Crafting a Winning Sports Betting Model
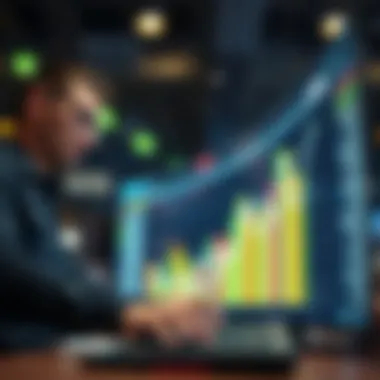
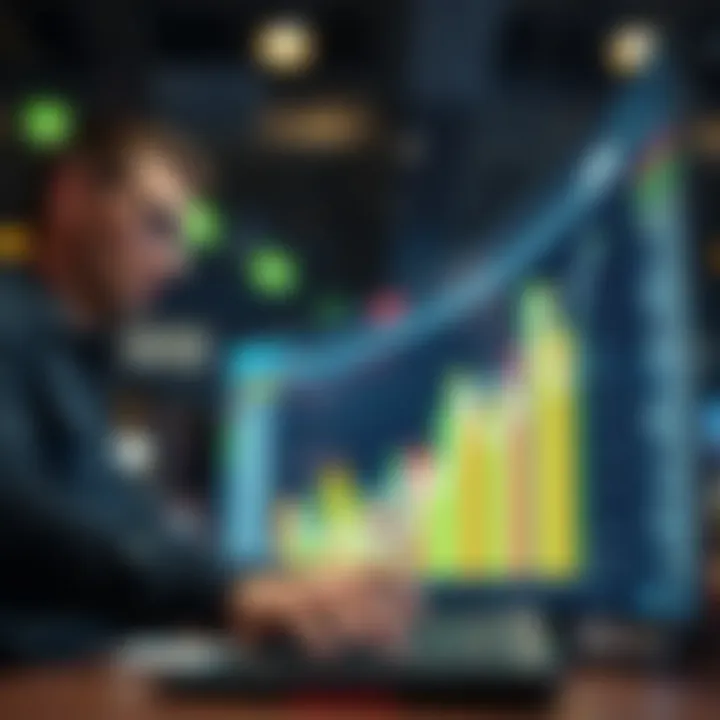
Intro
Creating a successful sports betting model isn't just about picking the teams with the best odds or the players who can kick the ball straight. The landscape is complex, woven with intricate patterns that can easily baffle even seasoned gamblers. A well-structured model can mean the difference between winning and losing, and without a robust foundation, you're essentially betting in the dark.
In this guide, we’ll dive deep into articulating elements that make a betting model effective, ensuring you grasp the importance of each component. From understanding statistical foundations to employing practical applications, the goal is to equip ambitious bettors with the tools and insights needed to enhance their predictions. Throughout the article, we will also emphasize responsible gaming principles, acknowledging that even in the realm of statistics, understanding personal limits is key.
If you've ever felt overwhelmed by massive data sets or unsure about the right strategies to adopt, sit tight. You'll learn
- The essential components of a sports betting model
- Data collection techniques that deliver actionable insights
- Analysis methods that are user-friendly yet efficient
- Strategies to validate your model's effectiveness
- How to approach responsible gaming
By the end of this piece, you’ll have gained a more comprehensive understanding of what it takes to create an effective sports betting model, ultimately giving you a better shot at predicting outcomes with confidence.
Betting Strategies
When it comes to sports betting, knowing your strategy can be comparable to wielding a paintbrush in a gallery. Each stroke matters and can create an entirely different picture. Not all bettors are the same, hence it’s crucial to mold a strategy that fits your personal style and risk appetite.
Advanced Betting Techniques
In a world where traditional methods are often heralded as gospel, exploring advanced strategies could set you apart from the crowd. Techniques, such as value betting, rely heavily on discerning when the odds offered are greater than the probabilities of an event happening.
As a bettor, you need to develop a keen eye for identifying underestimated teams or players.
- Statistical Analysis: Digging into stats beyond what the bookies provide can open new doors. For instance, understanding variables like player fatigue or injury status can reveal advantages not immediately visible.
- Trend Analysis: Following long-term trends might help pinpoint patterns that are often overlooked. Changes in coaching staff or roster can influence team behavior and expected performance.
Example of Trend Analysis
Consider a scenario where Team A has consistently beaten Team B over the last five years during home games; despite injuries, if their historical win rate is significantly high, it may indicate a hidden value in betting.
Bankroll Management Tips
Managing your finances wisely is equally essential to your strategy. Your bankroll is your lifeline in the betting world, and treating it with respect will serve you well in the long run.
- Set your limits: Determine how much you're willing to risk in a specific period.
- Staking Plan: Determine a staking plan that corresponds to your comfort level. For example, a flat betting strategy involves betting a fixed amount regardless of perceived value. In contrast, a proportional system may encourage betting more when your bankroll rises.
"Success doesn’t come from what you do occasionally, it comes from what you do consistently." – Tony Robbins
The focus here is not merely to win; it’s about creating a sustainable method that’s built for the long haul. By melding strategy with careful management, your sports betting model can not only thrive but also evolve with your betting journey.
Understanding Sports Betting Models
When it comes to engaging in sports betting, having a well-crafted model can mean the difference between just throwing darts and hitting the bullseye. An effective sports betting model isn't just a fancy spreadsheet; it's a structured approach that pinnacles the art and science of making predictions in sports. Here, we will dissect the necessity of understanding sports betting models and touch upon their essential components, benefits, and considerations.
A sports betting model systematically crunches data to provide insights on outcomes. Think of it as a sophisticated weather forecast but for the world of sports. Just as meteorologists use vast amounts of historical and real-time data to predict rain or shine, bettors leverage statistics, trends, and numerous variables to gauge the likeliness of a team's victory. No stone is left unturned in the pursuit of accuracy, which is crucial because mistakes can be costly. The more robust the model, the higher the chances of fostering successful betting strategies.
Definition of a Sports Betting Model
A sports betting model can be defined as a quantitative framework that aids bettors in assessing and predicting the results of sporting events. This model draws on a variety of statistical methods and data sources, striving to generate odds that reflect the true likelihood of a team's success.
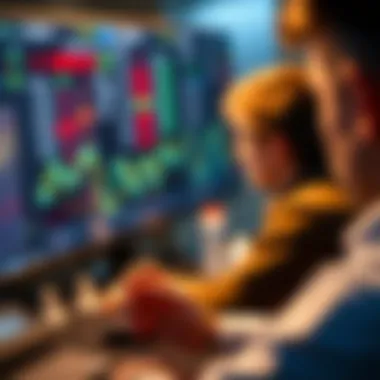
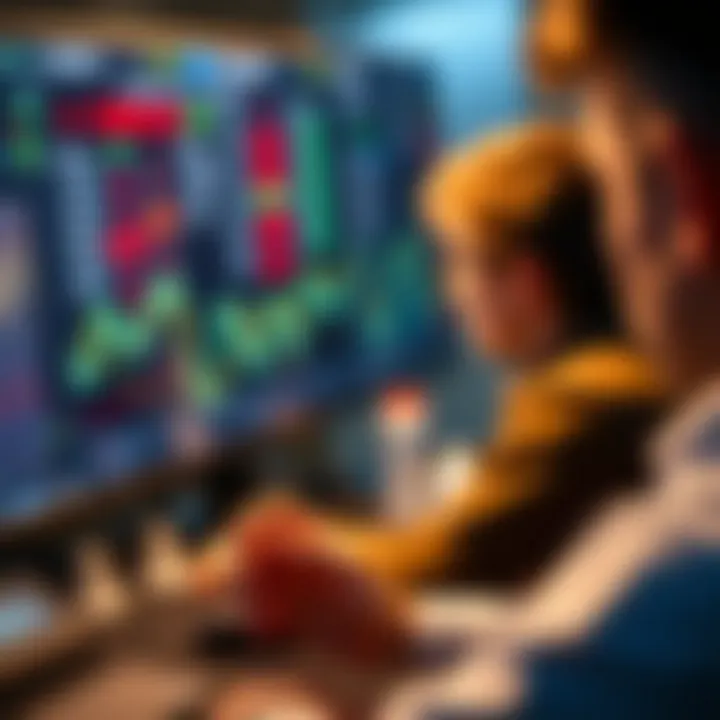
In essence, these models take inputs like player stats, weather conditions, historical match results, and even advanced metrics, like player efficiency ratings, and transform them into probabilities. By employing such a structured methodology, gamblers can minimize gut feelings and focus on objective analysis. Here’s a breakdown of what a solid betting model often includes:
- Historical performance data of teams or players
- Injuries and player transfers
- Venue conditions (e.g., home ground advantage)
- Weather forecasts for outdoor matches
The definition of a sports betting model doesn’t stop at data processing; it’s also about adjusting and interpreting this data in a way that it serves your betting objectives.
The Importance of Predictive Models in Betting
Predictive models have a significant role in the betting landscape. They not only help in estimating outcomes but also provide a strategic edge over competitors. Understanding their importance boils down to a few key points:
- Accuracy: The primary reason for using these models is to improve the accuracy of bets. Statistical analysis leads to more reliable predictions.
- Risk Management: Knowing when to put your money where your mouth is becomes pivotal. Predictive modeling helps in identifying high-value bets while steering clear of dangerous ones.
- Edge Over Bookmakers: Bookmakers usually have algorithms and proprietary models, but bettor-created models can help level the playing field.
"In sports betting, information is your closest ally. Models transform data into actionable insights."
Moreover, predictive models enable players to adapt their strategies dynamically. Just like a seasoned chess player who adjusts their tactics based on the opponent’s moves, bettors can alter their betting behavior as new data comes in or as conditions change. This flexibility can be a game changer in a market as volatile as sports betting.
In summary, understanding sports betting models is crucial for anyone looking to navigate the intricacies of betting efficiently. From laying the groundwork through proper definitions to recognizing the critical impact of predictive analytics, every aspect contributes to an enriched betting experience.
Fundamental Components of a Betting Model
Crafting an effective sports betting model is not merely a game of chance; it's about using a systematic approach to analyze and predict outcomes. At the heart of this endeavor are the fundamental components that underpin the model's framework. Understanding these components can lead to more informed betting decisions and potentially higher returns. By setting a solid foundation, bettors can navigate the complex world of sports betting with more confidence.
Key Variables to Consider
When attempting to develop a reliable sports betting model, a bettor ought to consider various key variables. These variables help in shaping the predictive nature of the model. Here are crucial aspects to keep in mind:
- Team Performance: Statistics such as win-loss record, points scored, and defensive metrics are vital. For instance, if a basketball team has consistently high offensive efficiency paired with strong defensive rebounds, this may signal a good betting opportunity.
- Player Statistics: Individual player stats can crucially impact the outcome of a game. Look into metrics like shooting percentage in basketball or rushing yards in football. An injured player can swing the odds, so stay alert to injury reports, trades, and player form.
- Location of the Game: Home-field advantage is a real phenomenon in sports. Home teams tend to perform better, often for reasons like familiar surroundings and fan support. Thus, whether a game is played at home or away may serve as a significant variable in your model.
- Coaching and Strategy: Different coaches employ various strategies which can yield varying outcomes. Understanding a team’s playing style helps bettors anticipate how a game might unfold, making this consideration crucial.
- Weather Conditions: Especially in outdoor sports, the weather can play an important role. Wind, rain, or snow can affect player performance, which may not be reflected in typical statistics but can significantly impact the final score.
Incorporating these variables into your model can enhance its predictive accuracy. Without them, your model risks being as unreliable as a coin toss.
Types of Data Sources
Data is the lifeblood of any betting model. The types of data sources you choose to leverage will greatly influence the insights and predictions you can draw. Below are some common sources of data that can enhance your model substantially:
- Official Statistics: Websites like ESPN or the league's official page offer a wealth of up-to-date statistical information, from player stats to historical performance records. These are reliable sources for current and comprehensive data.
- Publicly Available Databases: Platforms like the Sports Reference family of sites provide extensive statistical data, often for free. These databases can help in establishing a historical context for your model.
- Betting Markets: Odds from various betting platforms reflect the collective wisdom and information of the market. Monitoring how odds fluctuate can help you gauge public perception and sentiment surrounding certain teams or players. In turn, this can provide insight into potential value bets.
- Social Media and News: While it can be a bit hit-or-miss, social media platforms like Twitter can offer real-time updates about player injuries, trades, and other relevant news. Additionally, following sports analysts or journalists can provide nuanced perspectives on upcoming games.
- Custom Data Sets: Some bettors choose to develop their own data collection strategies, perhaps using web scraping tools to gather information from forums or other non-traditional sources. While this can be tedious, the unique insights drawn from these datasets may offer an edge.
The integrity and relevance of the data you employ can make or break your betting model. Without good data, even the best analysis methods are futile. Always ensure your sources are reputable and your data is current.
In the world of sports betting, relying on outdated or misinformed data is akin to betting on a race without knowing the horses; it’s almost sure to end in disappointment.
Data Collection Techniques
In the realm of sports betting, the accuracy of your predictions is heavily reliant on how well you gather and analyze data. The significance of data collection techniques cannot be overstated, as they set the foundation for your betting model's effectiveness. Engaging in meticulous data collection ensures that you have relevant information at hand, steering clear of misunderstandings or misplaced assumptions that could lead to realizations of losses.
When it comes to sports betting, your model can only be as good as the data it analyzes. Robust models hinge on the use of precise, timely, and comprehensive information. Let's dive into the various ways to ensure that your data collection methods are spot-on and can bolster the performance of your betting strategies.
Identifying Reliable Data Sources
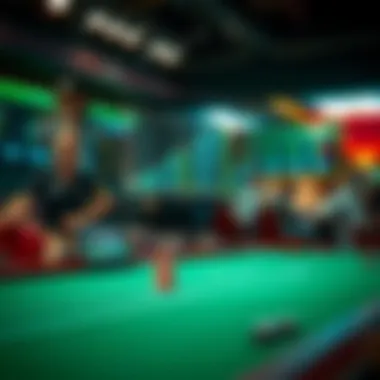
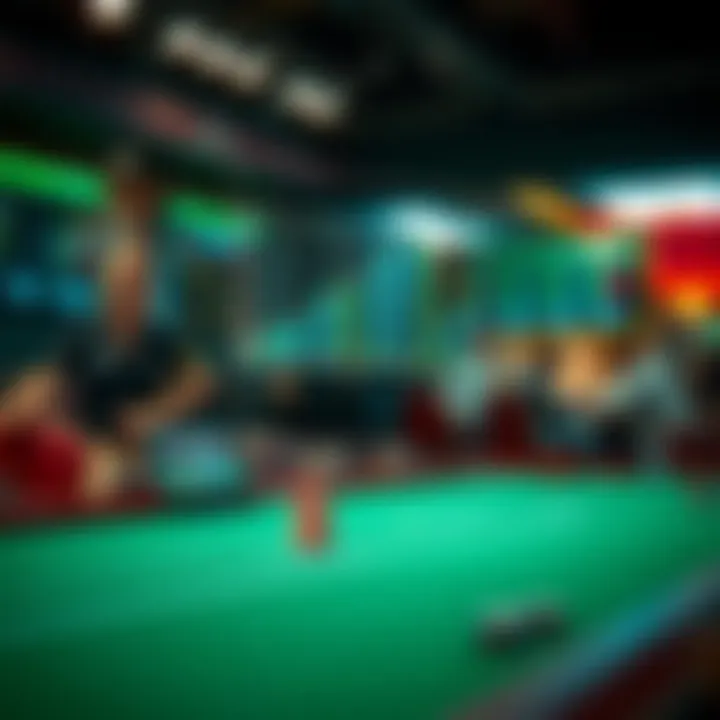
The first step in the data collection process is pinpointing reliable data sources. High-quality and trustworthy data underpins all analytical processes essential in sports betting. Rather than gathering information from any old corner of the internet, betting enthusiasts should look for databases, sports analytics websites, and other reputable platforms.
Some well-regarded sources include:
- ESPN – Wideranging statistics and player analysis
- Sports Reference – Detailed historical performance data
- Statista – Vital market statistics and trends on betting and sports
- National Institute for Sports Analytics – Academic articles and statistical methodologies
Additionally, forums like Reddit can offer insights, community discussions, and tips on where to find useful data. Just keep a lookout, as sometimes the opinions there might not be as reliable as the data. Consider cross-checking among various sources to validate the authenticity of the information you gather.
Automating Data Collection
Once you've identified viable data sources, it's time to discuss automating data collection. Automation can greatly enhance efficiency, allowing you to spend your time analyzing rather than scrambling to collect numbers. You can utilize various software tools and APIs to pull data without your constant oversight.
Scripts can be written in programming languages like Python to scrape data from websites or pull specific information via APIs. Some popular libraries are:
- Beautiful Soup – For web scraping
- Pandas – For data manipulation and analysis
Take note: while automation streamlines the process, ensure you maintain an ethical approach. Always abide by the terms of service of the websites you are scraping from, or you might find yourself in hot water.
Data Cleansing and Preparation
After collecting data, it’s paramount to enter the stage of data cleansing and preparation. Raw data often contains inconsistencies, errors, or missing values which can skew your findings. A clean dataset is the backbone of any successful statistical analysis.
Here’s how to go about it:
- Remove duplicates: Eliminate any repeated entries that can affect your analysis
- Fix inconsistencies: Make sure names and terminologies are uniform throughout the dataset
- Handle missing values: Decide whether to fill in missing data (with averages, for instance) or to remove those entries altogether
- Format data properly: Convert numerical values into a suitable format for your analytical model
Investing time in this stage pays off in the long run because a clean dataset diminishes the likelihood of erroneous predictions. The cleaner your data, the more substantial insights you can draw from your analyses.
The effectiveness of your betting model hinges on the quality of the data: Garbage in, garbage out.
Utilizing effective data collection techniques positions you to build a sports betting model laden with integrity and potential. As you gather, automate, and cleanse data, you empower your betting strategies, putting you a step ahead in the arena of sports analytics.
Statistical Methods for Model Building
In the realm of sports betting, statistical methods are not just a fancy add-on; they are the backbone of any reputable betting model. Their importance cannot be overstated as they provide a structured way to analyze performance data, derive valuable insights, and make informed predictions. Betting without a solid statistical foundation is akin to sailing without a compass; you could end up anywhere, and it’s not usually a good place.
Understanding the statistical methods applicable in this domain enhances your ability to evaluate risks and improve your odds for strategic outcomes. Let's delve deeper into the specific statistical techniques that form the core of effective sports betting models.
Descriptive Statistics
Descriptive statistics serve as a foundational element in model building, giving insights into how various elements of sports performance may behave over time. This basic form of statistics summarizes data to provide insights through figures like means, medians, modes, and standard deviations. For instance:
- Means help you identify the average score of a team, which gives you a preliminary glimpse into their overall capability.
- Standard deviations highlight variability within game scores, allowing you to identify inconsistency in team or player performance.
By understanding these metrics, you can spot trends or anomalies that might influence your betting decisions. It's not just about numbers; it’s about what those numbers represent in the context of the sports betting landscape.
Inferential Statistics
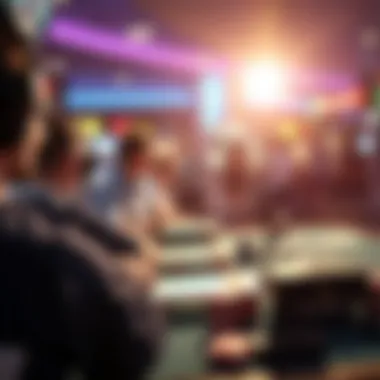
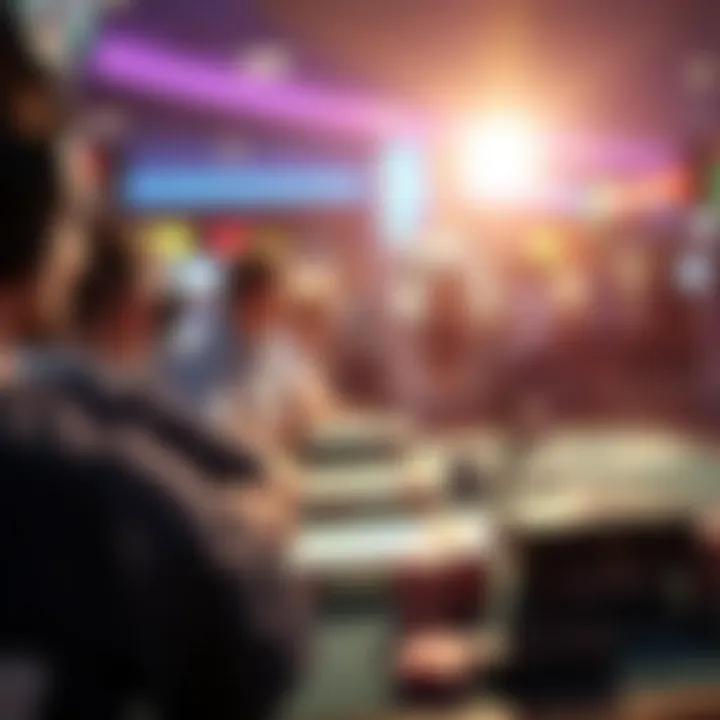
Inferential statistics takes you a step further by allowing you to make predictions and draw conclusions from a sample of data. This is particularly useful when full data sets aren't available. Techniques like hypothesis testing, confidence intervals, and statistical inference empower you to:
- Estimate the potential outcomes of matches based on historical performance.
- Gauge the reliability of your predictions, understanding if the data you have sufficiently represents the larger scenario in question.
For example, if you want to predict a basketball game outcome based on past performances, inferential statistics lets you extend conclusions from your sample to the general population of games, sharpening your betting strategy.
Regression Analysis
When it comes to discerning relationships between variables, regression analysis is the go-to method. This technique helps you understand how different factors, such as player injuries, historical performance against certain teams, or even weather conditions, impact the results of a game. By applying regression models, you can:
- Determine which factors are statistically significant when it comes to predicting outcomes.
- Build a predictive equation that works like a formula to forecast possible results.
In essence, regression analysis transforms raw data into a practical tool that can guide your bets with greater accuracy.
Machine Learning Applications
As technology advances, incorporating machine learning into betting models is becoming more commonplace. This involves using algorithms that learn from historical data to make better predictions over time. With machine learning, you can:
- Process large datasets more efficiently.
- Adapt your models dynamically as new data comes in, refining your predictions with minimal manual input.
Some common applications include decision trees, neural networks, and ensemble methods, which all provide a nuanced look at factors affecting sports outcomes. This method holds immense potential for making decisions that could tilt the odds in your favor.
*"In betting, just like in life, the more data you have, the less you leave to chance."
By utilizing these various statistical methods in your betting model, you create a robust framework that not only aids in predictions but also permits continuous learning and adaptation as new data and insights become available. The synergy among descriptive statistics, inferential reasoning, regression methodologies, and modern machine learning applications can delineate your approach to betting and secure a more educated edge in an often unpredictable domain.
Model Testing and Validation
Model testing and validation form the backbone of any effective sports betting model. Without proper testing, you risk having a model that delivers inconsistent results, leading to poor betting decisions. This section zeroes in on the importance of rigorously examining your model's predictions and its overall reliability. Think of it as tuning a finely crafted instrument; if you don’t validate your settings, you may hit the wrong notes when it matters most.
Backtesting Techniques
Backtesting is a crucial technique for any serious bettor looking to evaluate their model's predictive power. Essentially, it involves testing the model using historical data to see how it would have performed in real-market conditions. Here are a few things to consider:
- Historical Relevance: Use historical data that represents the types of conditions your model will be applied. Whether it’s a specific team’s performance in certain weather conditions or the impact of key player injuries, the data must be both relevant and comprehensive.
- Robust Sample Size: A small sample can lead to misleading conclusions. Aim for a sample size that encompasses multiple seasons or a broader time frame to get a clearer picture.
- Out-of-Sample Testing: Once your model performs well on historical data, it’s vital to test it on out-of-sample data to gauge its applicability to future events. This helps in identifying overfitting, which is when a model performs well on training data but fails to generalize.
To conduct backtesting, you might want to employ software tools designed for this purpose. A common tool used is R with libraries such as . A basic structure for backtesting with R might look like this: r
Example R Code for Backtesting
library(quantstrat)
basic setup
declareParams()
build strategy
strategy("sample_strategy")
test against historical data
testResults - applyStrategy(strategy, data=snapshotData)